Keynotes
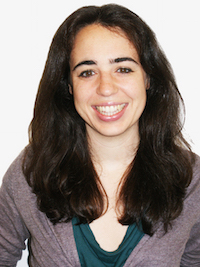
Miriam Fernandez, Knowledge Media Institute, Open University (UK)
Artificial Intelligence for policing
In this talk we provide an overview of how Artificial Intelligence and technology development can contribute to existing policing practices. In particular, we will discuss three different projects conducted in the context of the Centre of Policing Research and Learning of the Open University (http://centre-for-policing.open.ac.uk). The first project, policing engagement via social media, provides an overview of how police forces in the UK make use of social media to communicate with the public, and what attracts citizens to engage with social media policing content. The second project, detecting grooming behaviour on social media, describes the problem of child grooming online, and our proposed approach to automatically identify the different stages of grooming behaviour from online content. The third project, radicalisation detection on social media targets the problem of online radicalisation, where individuals are introduced to extreme views, primarily through the use of social media. Within this context our work focuses on the detection of pro-ISIS stances and on the understanding of the processes that influence the adoption of radicalised behaviour. This talk will explore the opportunities and limitations of using AI techniques, and particularly social media analysis methods, to introduce change within the policing landscape, with a greater emphasis on evidence-based practice.Speaker Bio
Dr Miriam Fernandez is a Research Fellow at the Knowledge Media Institute (KMi), Open University, and a senior member of the Social Semantics and Web Science group. Before joining KMi, she was research associate at Universidad Autonoma de Madrid, Spain and software engineer (internship) at Google Zurich, Switzerland. Her research is at the intersection of the Web Science (WS) and Semantic Web communities (SW), where she has contributed with more than 100 peer-reviewed articles in various leading conferences and journals. She has extensive expertise in leading EU and national projects. She frequently participates in organising committees and editorial boards of the top SW and WS conferences, recently being program co-chair of the International Semantic Web conference in 2017, and serving as editor for the Journal of Web Semantics.
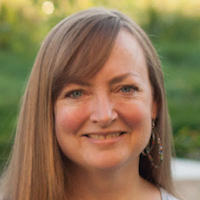
Vanessa Murdock, Amazon (US)
Cutting the Cruft from the Toasts
Notifications appear on the lock screen of a mobile phone, hovering at the top of an open app, as pop-ups (``Toast" notifications) on a desktop device or gaming console, posing a significant distraction to users. In our data, the median user receives five notifications per hour on mobile and desktop devices, which is a distraction every 12 minutes. At the 75th percentile, users receive 17 notifications per hour - one every 3.5 minutes. While users receive many notifications, they engage with very few. Toast notifications in our sample have less than a 5% click-through rate, and the most prolific sources of notifications often have the lowest engagement. In this paper we present a large-scale analysis of user engagement with notifications on mobile devices and on the desktop. Our study includes a large sample of billions of Toast and message center notifications sampled over a 2 month period, from hundreds of millions of users. Based on our observations, we show that we can improve the click-through rate on Toast notifications from 5% to 35% and on notifications in a message center from 29% to 85%. If we instead rank the notifications in the message center, we improve the precision at rank one from 29% to 49%.Speaker Bio
Vanessa Murdock is a Science Manager at Amazon. Previously, she worked at Microsoft as Principal Applied Researcher in Cortana Research, and before that she led a research team that developed ML techniques for advertising, device fingerprinting, and leveraging social media to improve local search. Prior to Microsoft, Murdock worked at Yahoo! Research in Barcelona, Spain where she led the Geographic Context and Experience Group, doing research on topics related to geographic information retrieval and user-generated content. She has been awarded 15 patents, and has more than 30 patent applications pending, resulting in a Master Inventor Award from Yahoo! (2012). She received the OAA Award for Outstanding Achievement by a Young Alum from the University of Massachusetts in 2014. She has more than 50 publications in the area of Information Retrieval. Murdock received a Ph.D. in Computer Science from the University of Massachusetts Amherst in 2006.
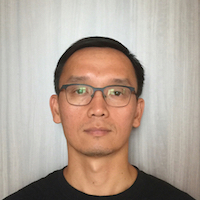
Hung Q Ngo, Relational AI Inc. (US)
Worst case optimal join algorithms: techniques, results, and open problems
Speaker Bio
Hung Q. Ngo was a professor at the State University of New York at Buffalo from 2001 to 2015. From 2015, he started working for a couple of startups building datalog and data analytic engines: LogicBlox and RelationalAI. His current research and development interests include the design, analysis, and implementation of in-database computation algorithms. These algorithms cover typical logic and statistical query optimization. He received a CAREER award, a COCOON 2008 best paper award, PODS 2012 and PODS 2016 best paper awards.
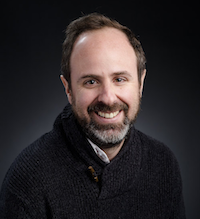
Pablo Barceló, University of Chile (CL)
A Theoretical View on Reverse Engineering Problems for Database Query Languages
Speaker Bio
Pablo Barceló is an Associate Professor in the Department of Computer Science at the University of Chile. He received his PhD from the University of Toronto in 2006. His main research interest are in the areas of databases and logic in computer science, where he has contributed with over 40 technical papers in major conferences and journals. He has also been an invited tutorial speaker at ACM PODS 2013. He is a member of the editorial board of Logical Methods in Computer Science and a former editor of the Database Principles Column of the SIGMOD Record. During 2019 he will be chairing the International Conference on Database Theory (ICDT).